The Ultimate Guide to Data Annotation Platforms
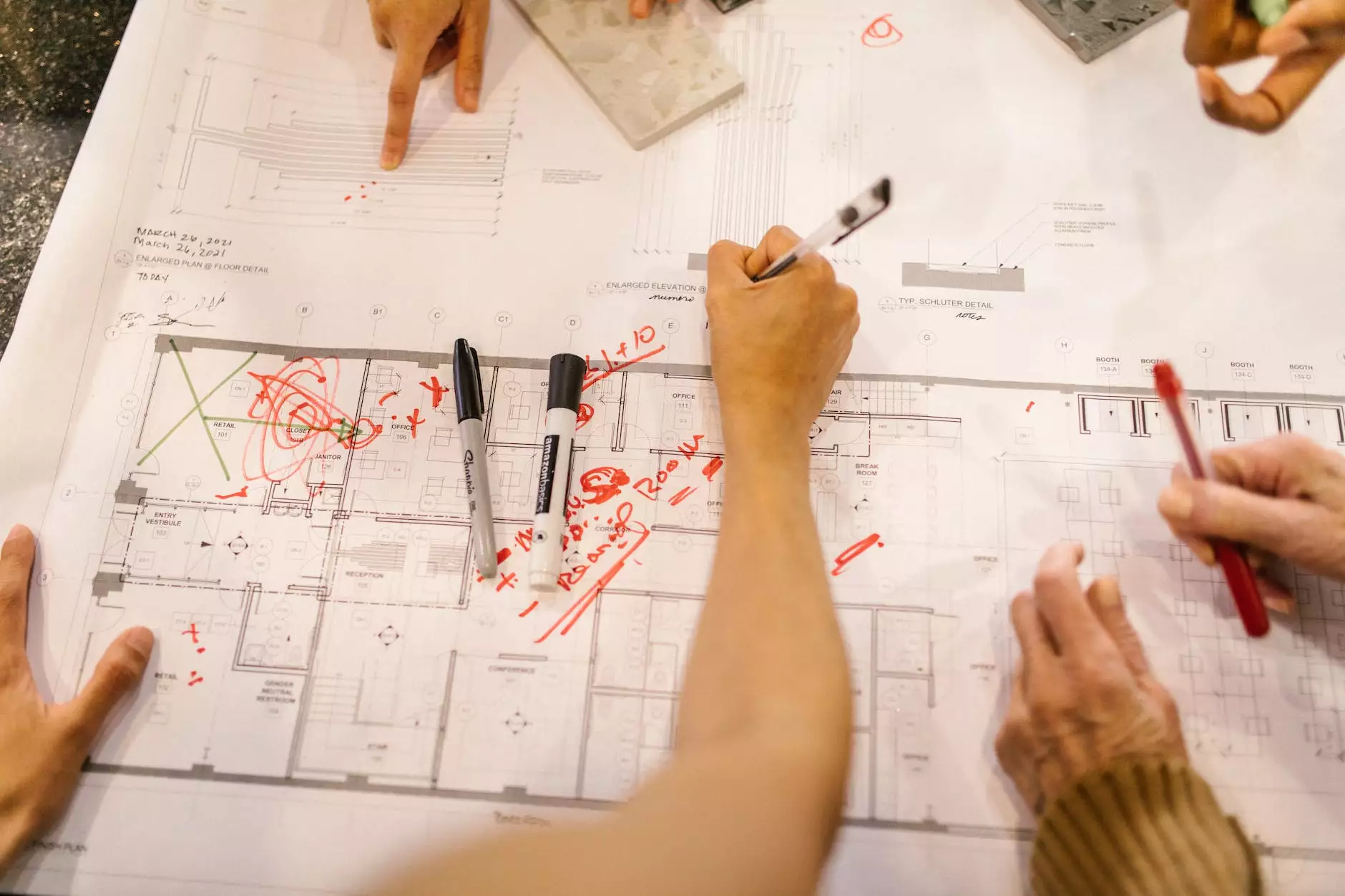
In today’s rapidly evolving technological landscape, data annotation platforms play a pivotal role in the growth and accuracy of artificial intelligence (AI) and machine learning (ML) applications. As businesses strive to harness the power of AI, understanding the intricacies of these platforms becomes essential. In this comprehensive guide, we will explore the significance of data annotation, its impact on various sectors, and how selecting the right platform can revolutionize your business operations.
1. Understanding Data Annotation
Data annotation refers to the process of labeling data to make it comprehensible for algorithms. This practice is critical in training AI systems, allowing them to recognize patterns and make decisions based on vast amounts of information.
1.1 The Importance of Data Annotation
- Quality Training Data: High-quality annotated data ensures that AI models can learn accurately, improving their performance.
- Enhanced Accuracy: Properly labeled data leads to better prediction capabilities and overall reliability of AI systems.
- Speeding Up Development: Annotated datasets streamline the model training process, reducing development time.
- Adaptability: Different industries require various types of data annotation, from images to text, highlighting the need for customizable solutions.
2. Types of Data Annotation
Data can be annotated in several formats, each serving unique purposes across diverse industries. Let's delve into some of the common types of data annotation you might encounter:
2.1 Image Annotation
Image annotation involves labeling images for computer vision technologies. This includes tasks such as:
- Object Detection: Identifying and labeling objects within an image.
- Semantic Segmentation: Dividing images into segments and labeling them.
- Image Classification: Categorizing images into predefined classes.
2.2 Text Annotation
Text annotation is critical for natural language processing (NLP) applications. It includes:
- Named Entity Recognition (NER): Identifying and categorizing entities in the text (e.g., names, dates).
- Sentiment Analysis: Assigning sentiment labels to texts based on tone and emotion.
- Part-of-Speech Tagging: Labeling words in a sentence based on their grammatical role.
3. Business Applications of Data Annotation Platforms
Data annotation platforms have become invaluable across various industries. Here’s how they are transforming businesses:
3.1 Healthcare
In the healthcare sector, data annotation is used for:
- Medical Imaging: Annotating X-rays, MRIs, and CT scans for improved diagnostic accuracy.
- Patient Records: Organizing and categorizing patient data for enhanced healthcare delivery.
3.2 Automotive
The automotive industry relies on data annotation for:
- Autonomous Vehicles: Labeling data from LIDAR and camera input helps in training self-driving algorithms.
- Driver Assistance Systems: Annotating scenarios to enhance safety features in vehicles.
3.3 Retail and E-commerce
In retail, data annotation aids in:
- Customer Insights: Analyzing customer behavior and preferences through annotated data.
- Visual Search: Enabling image recognition features for better product discovery.
4. Choosing the Right Data Annotation Platform
Selecting a data annotation platform that suits your business needs is crucial. Here are some factors to consider:
4.1 User-Friendly Interface
A straightforward and intuitive interface can significantly enhance the efficiency of your team. Look for platforms that offer an easy-to-navigate design, allowing both technical and non-technical users to contribute effectively.
4.2 Scalability
As your data needs grow, so should your platform. Choose a solution that can scale with your business and handle increasing volumes of data without compromising performance.
4.3 Quality Control
Ensuring the accuracy of annotated data is paramount. Look for platforms with built-in quality control measures, such as review systems and real-time feedback loops.
4.4 Turnaround Time
Speed is critical in today’s fast-paced environment. Evaluate platforms based on their ability to deliver timely annotations without sacrificing quality.
4.5 Integration Capabilities
Your chosen platform should seamlessly integrate with existing tools and workflows. Check for APIs and compatibility with machine learning frameworks used in your organization.
5. Conclusion
In conclusion, data annotation platforms are vital to the success of AI and machine learning initiatives across various industries. As businesses increasingly rely on AI-driven decisions, investing in a robust data annotation solution becomes imperative. By understanding the types of annotations, their applications, and how to select the right platform, organizations can harness the full potential of data and remain competitive in the ever-evolving market.
At Keymakr, we recognize the importance of adaptable, high-quality data annotation services tailored to your unique business needs. Partnering with us ensures access to a reliable and efficient data annotation platform that can elevate your AI capabilities and drive your business forward.