Understanding Bounding Boxes in Data Annotation
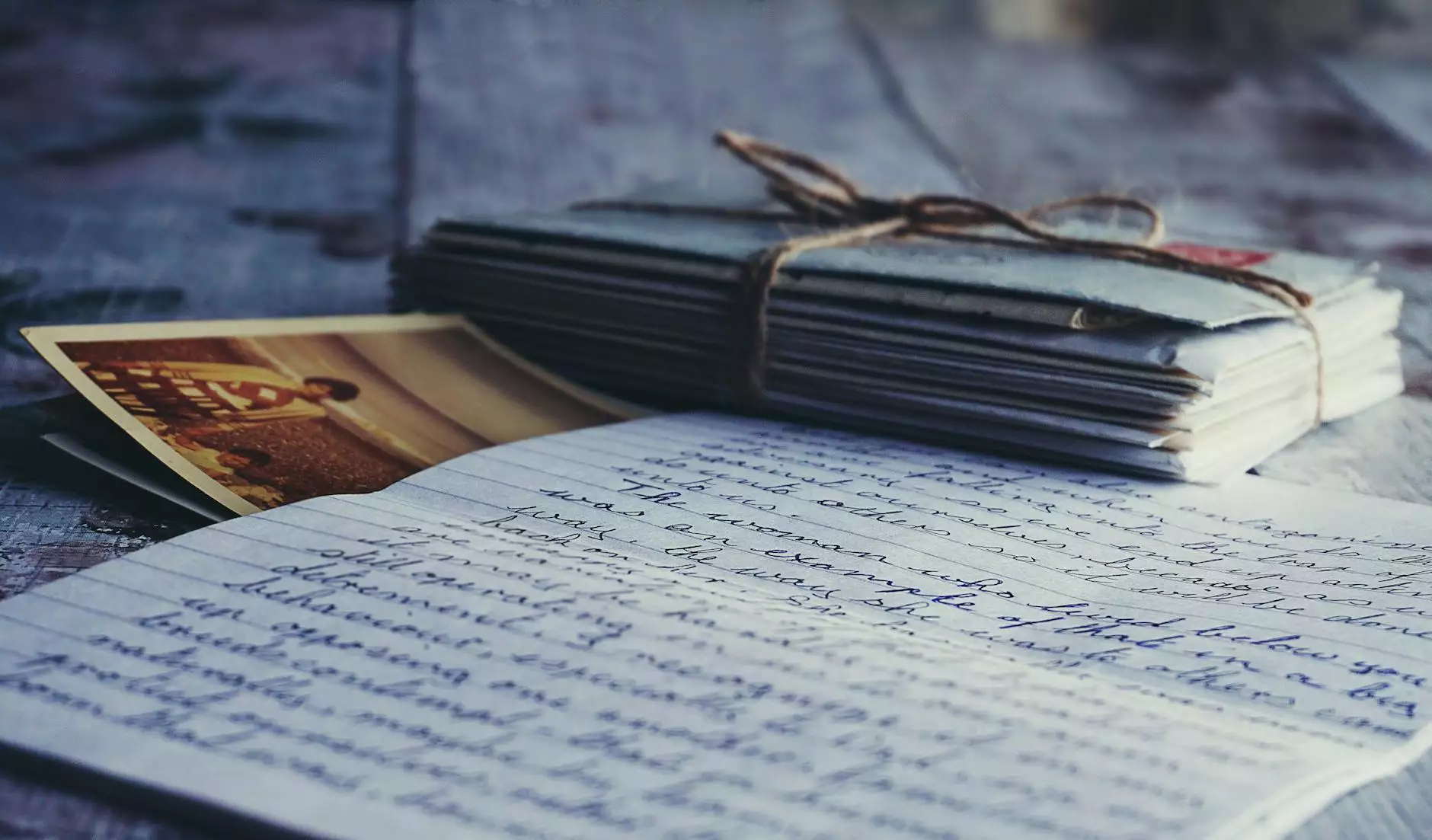
In the ever-evolving landscape of data annotation, bounding boxes play a crucial role. They are used in numerous applications, particularly in the field of computer vision. As businesses strive to leverage technology for data-driven decisions, understanding and implementing effective data annotation tools becomes essential.
What are Bounding Boxes?
Bounding boxes are rectangular boxes that are drawn around objects in an image or a video frame. They serve as a basic yet pivotal procedure in visual data annotation. The bounding box defines the object's location and helps machine learning models accurately identify and classify objects within an image.
Why Use Bounding Boxes?
The utilization of bounding boxes in data annotation is prevalent due to several reasons:
- Object Localization: Bounding boxes help in locating objects within images, enabling sophisticated analysis and recognition.
- Model Training: They are integral in training machine learning algorithms to distinguish between different objects or classes effectively.
- Efficiency: Providing a defined area for machines to process reduces errors in object detection, increasing the overall efficiency of AI applications.
- Versatility: Applicable across various industries such as retail, security, automotive, and healthcare, making them universally important.
Applications of Bounding Boxes
The practical applications of bounding boxes are vast and impact numerous sectors:
1. Autonomous Vehicles
In the realm of self-driving cars, bounding boxes are fundamental for detecting pedestrians, vehicles, and road signs. They assist the vehicle's AI in understanding its environment for safer navigation.
2. Retail and Inventory Management
Retailers utilize bounding boxes to monitor stock levels and manage inventory effectively by tracking the locations and amounts of various products on shelves through visual data analysis.
3. Security and Surveillance
In security systems, bounding boxes help in identifying suspicious activities in surveillance footage, thereby enhancing safety and security measures in public spaces.
4. Medical Imaging
In the healthcare sector, bounding boxes are used in medical imaging to identify anomalies, such as tumors in MRI scans. This helps in early diagnosis and improves patient outcomes.
The Process of Creating Bounding Boxes
The creation of bounding boxes involves a systematic approach that can be broken down into the following steps:
Step 1: Data Collection
The first step is gathering high-quality images or videos that represent the data to be annotated. Quality is crucial for the effectiveness of the machine learning models.
Step 2: Annotation
Using a data annotation tool, annotators manually draw bounding boxes around the objects of interest in each image. This action requires precision to ensure that the box accurately encloses the entire object.
Step 3: Quality Control
After the initial annotation, it is essential to conduct quality control checks. This process involves reviewing the annotated images to rectify any inaccuracies or discrepancies in the bounding boxes.
Step 4: Model Training
The final step is to use the annotated data for training machine learning models. The success of these models heavily relies on the quality of the bounding boxes, making this step critical.
Challenges in Bounding Box Annotation
While bounding box annotation is fundamental, it does come with challenges that businesses need to be aware of:
- Complex Objects: Objects that are occluded or overlapping can complicate the annotation process.
- Ambiguity: Some objects may not have clear boundaries, leading to uncertainty in how to draw the bounding box.
- Time-Consuming: Manual annotation can be tedious and time-consuming, impacting project timelines.
- Consistency: Maintaining consistency across multiple annotators is crucial to ensure quality but can be challenging.
Utilizing Data Annotation Platforms
To address the challenges of bounding box annotation, businesses can leverage advanced data annotation platforms like KeyLabs.ai. These platforms offer several benefits:
1. Automated Annotation Tools
Many modern data annotation tools incorporate AI to automate portions of the annotation process, significantly reducing time and effort required for bounding box creation.
2. High Scalability
Annotation platforms are designed to handle large volumes of data efficiently, making them ideal for businesses with substantial datasets.
3. Collaborative Features
These platforms often include collaborative tools that allow teams to work together, facilitating better consistency and quality control during the annotation process.
4. Customizable Workflows
Businesses can customize their workflows to tailor the annotation process to their specific needs, enhancing overall productivity.
Conclusion
In conclusion, bounding boxes are a vital component of data annotation that drives machine learning and AI advancements across various industries. By employing effective data annotation tools and platforms, businesses can streamline their data preparation processes, leading to improved machine learning outcomes.
Understanding and utilizing bounding boxes correctly can foster innovation and efficiency in business operations, making it an essential skill in today’s data-centric world. For businesses looking to elevate their data annotation processes, exploring cutting-edge solutions like KeyLabs.ai can provide significant advantages in the competitive landscape.